The problems with big data
Businesses are fixated with big data, but Aaran Fronda thinks they should exercise a little more caution
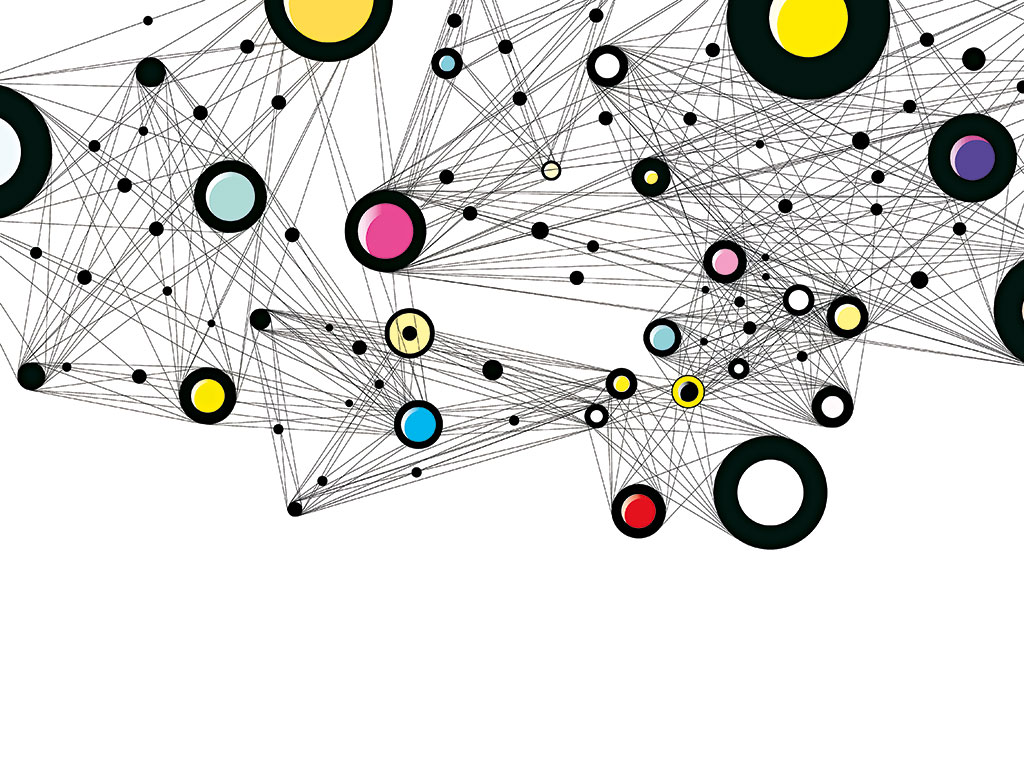
Companies such as OkCupid and Google have been keen to use big data to ascertain information about their customers. But in spite of its ability to uncover patterns, big data does not always explain the reasons why people do things
It’s not just the government that is interested in our data. Big business has grown obsessed with it too. While they may lack the authority of the NSA to pilfer our most personal information, there is still plenty of it for companies to get their hands on. Personal information is freely given away during the course of our daily delvings into the internet.
But because we give up this information of our own accord via Google searches, Twitter posts and Facebook status updates, economist and journalist Tim Hartford prefers to distinguish these datasets by labelling them ‘found data’. The reason businesses wish to access that which most view as useless is that they believe it helps them to learn more about us: the consumer. The thinking behind this massive mining is simple: the more a company knows about consumers’ habits, the easier it is to create and market products they will be willing to buy. But just how useful is big data and does it have any limitations?
In his 2014 Significance Lecture for the Royal Statistical Society, titled ‘The Big Data Trap’, Hartford discussed the pitfalls of putting too much faith in what can be gleaned from the masses of found data that is continually being collected. He begins the talk with reference to his friend Dan Ariely, a professor of psychology and behavioural economics at Duke University, who draws comparisons between businesses’ fixation with big data and that of teenage sex: “Everyone is talking about it, no one really knows how to do it, everyone claims they are doing it when they talk to other people, [and] everyone assumes everyone else is doing it.” The point Ariely is making is that, while there is a lot to be discovered through big data analytics when applied properly, many companies are just joining in on the latest craze, and, like adolescents, have no idea what they are doing in the data department.
Theory free
A great example of a business dipping into the world of big data, only to discover it does not have a clue why it bothered in the first place, is the online dating site OkCupid. Those familiar with its mobile app will be aware that, once an account has been set up and a biography created, it quickly prompts users to disseminate further personal information by asking them a wide array of multiple choice questions. These may relate to serious lifestyle choices (such as whether or not you smoke) to more arbitrary lines of questioning (like if you’re a lover of cats or dogs). The justification dating sites such as OkCupid give for intruding into your personal preferences is that these vast sums of data assist them in doing a better job of matching you with other users looking for love. But as the site’s founder, Christian Rudder, explains on his blog OkTrends, when it comes to big data “OkCupid doesn’t really know what it’s doing. Neither does any other website.”
That is not to say there are no great opportunities out there when big data analytics are used correctly. As Hartford explains in his lecture, Google was able to achieve incredible things through its use of massive datasets. He brings up the example of Google Flu Trends, which managed to track the spread of influenza across the US more effectively than the Centre for Disease Control and Prevention (CDC). Google’s findings, explains Hartford, were based on an analysis of search terms: “What the researchers announced in a paper in Nature five years ago was that, [by] simply analysing what people typed into Google, they could deduce where these seasonal flu cases were.” He notes that what makes this so remarkable is that Google was not only able to beat the CDC (their tracking only had a 24 hour delay, whereas it took the CDC a week to do the same thing), but that it was “theory-free”. In short, Google just ran the search terms users were putting into their search engine and let the algorithms do the rest.
Correlation is not causation
But using big data in this way has its limitations. Data-rich models like the ones employed by Google are great at finding patterns. In the case of tracking influenza outbreaks across the US by mapping where and when people searched criteria such as “flu symptoms” and “where is my nearest pharmacy”, Google was successful, but we should never become complacent about the results derived from this method. Never forget that correlation does not imply causation. Google fell foul of this. After successfully tracking flu outbreaks for a number of years, its big data model began to show signs of weakness, finding flu where there was none.
By just using search terms, the algorithms were treating all hits as hits, rather than as potential false flags. Just because people are searching flu symptoms, doesn’t necessarily mean they have it or are going to get it. There are countless examples available online of statistics being used to create obviously false connections, such as how the number of people who died falling out of wheelchairs correlates with the cost of potato chips. With massive datasets, these anomalies are not minimised: they are exacerbated.
The real problem with big data, which the Google Flu Trends example highlights, is that, while it is a very good tool for mapping what people are doing and where they are doing it, it is absolutely terrible at deciphering the reasons behind those actions. No matter how good we get at acquiring data, we can never get it all. Therefore the conclusions we derive from big data need to be looked at with an element of scepticism. Without a theory underpinning the cause of correlation, computer algorithms are just finding patterns in data and not much else. Big data is a clever new tool, but it is not a magic wand.